Abstract
The study aims to develop an eco-friendly solution for addressing contaminants in hospital wastewater. To achieve this, banana peel, a cost-effective and easily available agricultural waste, was chemically modified using sulfuric acid and sodium hydroxide. The hospital wastewater was analyzed using an Atomic Absorption Spectrometer to identify the presence of heavy metals. The physicochemical properties were measured and compared against the standards set by the World Health Organization (WHO). Scanning electron microscopy and Fourier-transform infrared spectroscopy were used to characterize the chemically modified banana peel. Batch adsorption was conducted with the wastewater using the prepared adsorbent. The effect of contact time, mixing rate, temperature, and adsorbent dosage was examined to know the removal efficiency of the adsorbent. The experimental design incorporates Taguchi OA design to optimize the process. The total heavy metals discovered in the wastewater were As, Cd, Fe, Cu, Ni, Cr, Pb, Mn and Zn. The percentage of Cd, Fe, Cu, Ni, Cr and Pb removed were 79.5%, 87.9%, 56.9%, 89.0%, 88.5% and 93.5%, respectively, at temperature, adsorbent dosage, mixing rate and contact time of 30 °C, 3 g, 700 rpm and 60 min, respectively. The physicochemical properties remain within the acceptable WHO range except the turbidity. At optimum point, the percentage removal of Cd, Fe, Cu, Ni, Cr, Pb and Zn were 64.3%, 80.5%, 51.4%, 82.3%, 72.4%, 86.6% and 72.1%, respectively; while, the conductivity, pH, TDS, and turbidity value were, respectively, 20.57 mS/cm, 6.8, 10.16 mg/l, and 86.31 NTU. Characterization revealed the existence of active functional groups on the adsorbent surface enhancing the hospital wastewater treatment and change in its morphological structure after the treatment was noticed. In conclusion, the modified banana peel was effective for the treatment of hospital wastewater.
Similar content being viewed by others
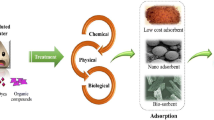
Avoid common mistakes on your manuscript.
Introduction
Pollution is a major problem in the world right now, it exists in majorly three forms namely; water pollution, air pollution and land pollution. Pollution may also exist in various forms such as thermal pollution, noise pollution and so on. Pollution is basically the release of harmful substances (pollutants) into the environment which causes a reduction in the quality of life in the environment (Perini et al. 2018). One of the main sources of land and water pollution is gotten from the hospital (Hocaoglu et al. 2021). The roles of hospitals in the life of human beings are invaluable due to their inestimable service provision in addressing complex health situations (Majumder et al. 2021). In doing this, a variety of chemicals (those needed for health-care facilities such as medical imaging and surgery; and therapeutic agents like antibiotics and disinfectants for curbing infectious diseases spreading) are utilized (Williams et al. 2018). All of these constitutes the wastewater generated from hospitals after the patients finished receiving medical attentions.
However, the presence of heavy metals, pharmaceuticals, X-ray contrast agents, antibiotics, disinfectants and substances with genotoxic, enteric pathogens, organic matter and cytotoxic activity in hospital wastewater make its presence in the environment (especially water and soil) to be dangerous to human health (Wielens Becker et al. 2020). These substances present in the hospital wastewater are usually from different hospital units/sources such as research activities, laboratory, diagnosis and patient medicine excretion (Paulus et al. 2019). The wrong disposal of hospital wastewater may result into surface water in which the wastewater substances may alter the aquatic ecosystem and obstruct the food chain. Thus, it is highly imperative to select a suitable treatment technique for hospital wastewater before disposal.
There are various methods in which the polluted substances in hospital wastewater can be removed. They are broadly classified as physical, chemical and biological methods of treatment (Gunatilake 2015). Adsorption, electrodialysis, reverse osmosis, evaporation, filtration, sedimentation, and flocculation are all physical treatment methods (Li et al. 2020; Popoola 2023b). Biological treatment include aerobic and anaerobic methods for the removal of pollutants from wastewater; while, some other methods like pre-ozonation, direct photolysis, photocatalysis and chlorination are also employed in the treatment of hospital wastewater (Mompelat et al. 2009; Renita et al. 2017). However, adsorption is the most common process used in the removal of trace organic pollutants from hospital wastewater, and it is one of the most important processes in the removal of pollutants from the environment (Popoola 2023a; Lala et al. 2023).
Some of the commonly used industrial adsorbents for the treatment of hospital wastewater are activated carbon, zeolites and carbon nanotubes (Li et al. 2020; Zekavat et al. 2020). However, there is need to generate low cost and environmental friendly adsorbents with high removal efficiency potential from agricultural wastes. By so doing, the nuisance caused by these agricultural wastes after the essential products harvest will drastically reduce while still using them as adsorbent sources for hospital wastewater treatment. To improve wastewater treatment, various adsorbents for the adsorption of various contaminants have been researched and developed (El-Sayed et al. 2014). Some of the recently used agro-wastes, from adsorbents were synthesized, to tackle environmental problems arising from the discharge of wastewater and other pollutants include chicken feather (Chen et al. 2019), peanut shell (Lee et al. 2019), grape pomace (Jin et al. 2020), onion skin waste (Yusuff et al. 2021b), spent coffee grounds (Oliveira et al. 2021), hull (Fita et al. 2023a, b) and Garcinia kola hull (Popoola 2023a). Many other studies have also proved adsorption as an effective water treatment method for the removal of heavy metals and contaminants from solution via the utilization of agrowastes as adsorbents (Adeyi et al. 2022, 2023a; b; Fita et al. 2023a, b; Popoola 2019a, b, 2020; Popoola et al. 2018, 2019, 2022; Yusuff et al. 2022).
The study by Gomes et al. (2020) revealed that banana peel amounting to 36 million tons is discharged as waste annually. This is supported by the inestimable health benefits of banana making people to eat it on a high rate. Also, the functional groups of carboxyl, hydroxyl and amine present in the banana peel have greatly influenced its application as a suitable adsorbent source for the removal of pollutants from solution (Leong 2018; Zaini et al. 2022). Studies have applied activated carbon from banana peel as adsorbent for the removal of heavy metals from solution (Arunakumara et al. 2013; Zhou et al. 2017; Afolabi et al. 2021). Limited studies have been conducted on its usage for the treatment of wastewater (Huzaisham et al. 2020). The study on the application of the chemically modified banana peel, using sulfuric acid and sodium hydroxide as a means of improving its adsorption capacity, for the treatment of hospital wastewater is still scanty.
This strengthens the novelty of this study coupled with the application of Taguchi OA design of Design Expert software (version 12.0.0) as the optimization tool for the hospital wastewater treatment process parameters (contact time, mixing rate, temperature and adsorbent dosage). The optimization of the hospital wastewater treatment process is imperative for operating cost reduction. The Taguchi design method is an optimization tool that offers a simple, efficient and systematic approach to optimizing design for performance, quality and cost. The use of the Taguchi design method in the development of adsorption processes can result in reduced process variability as well as the need for fewer time, reagents, and experimental runs (Yusuff 2019). For example, Yusuff et al. (2021a) used the Taguchi optimization method to optimize the adsorption of crystal violet dye by activated carbon from poultry litter. Also, Syed-Hassan et al. (2016) adopted this methodology to optimize activated carbon preparation from palm kernel shell and applied it for methane adsorption. The chemically modified banana peel used in this study before and after the hospital wastewater treatment was characterized via scanning electron microscope and Fourier-transform infrared spectroscopy.
Methodology
Materials
The research was carried out at the laboratories of Afe Babalola University Ado Ekiti’s Department of Chemical and Petroleum Engineering. The hospital wastewater sample was obtained from the Membrane Bioreactor of the Afe Babalola University, Ado-Ekiti (ABUAD) Multisystem Hospital’s treatment facility. The banana peels utilized as an adsorbent were sourced from the ABUAD Hostels’ domestic garbage. And finally, the chemicals used in the experiments were reagent/analytical grade and were used exactly as received.
Table 1 shows the list of chemicals used in achieving this research project.
Characterization of wastewater
The physiochemical properties and the heavy metals analysis was carried out to know the composition, and properties of the wastewater. The physiochemical properties measured and checked in the wastewater are; the turbidity, conductivity, salinity, pH and total dissolved solids (TDS). In carrying out the characterization, various machines and equipment such as turbidity meter, pH meter, conductivity meter and salinity/TDS pen were used. The characterization of the heavy metals was carried using Atomic Adsorption Spectrophotometer (Buck scientific model 210 VGP). The metals discovered are as follows; As, Cd, Fe, Cu, Ni, Cr, Pb, Mn, and Zn.
Preparation of adsorbent
The banana peels used was collected from domestic waste in hostels of Afe Babalola University Ado-Ekiti (ABUAD). After the collection of banana peels, it was then washed using distilled water to remove the adhering dirt, cut into smaller pieces and dried under the sun for an estimated period of 5 days. The sun dried banana peels were then placed in the oven for 4 h at 110 °C. The dried banana peels were blended and sieved using the mesh size of 425 µm. The procedure was adapted and modified from previous studies (Huzaisham et al. 2020; Mohamed et al. 2020, Arifiyana et al. 2021).
Chemical modification of adsorbent
45 g of the grinded banana peels was soaked in 600 mL of 0.4 mol L−1 H2SO4 for 24 h, after which the solution was neutralized by adding NaOH with concentration of 0.4 mol L−1. The modified banana peel was then filtered and rinsed with distilled water. The filtrate was then dried in the oven for 3 h at 80 °C to achieve a fine texture for adsorbent. The approach was revised and adapted from previous studies (Annadurai et al. 2003; Abdulfatai et al. 2013; Mohamed et al. 2020).
Experimental design
Using the Taguchi OA (Orthogonal array) design approach under factorial design, the adsorption process was optimized to be able to vary numerous parameters at a time which would be able to predict the optimum operating conditions for adsorption process. The Taguchi design used for the process was to vary four factors at a time with a design of 3-level-4-factor array so a total of 9 experimental run (L9) was conducted. Table 2 shows the factors considered and their respective levels.
The reaction contact time, adsorbent dosage, temperature, mixing rate were hand-picked as the independent variable for design; while, the pH was kept constant through the nine runs. Table 3 depicts the experimental design for the adsorption of pollutants from hospital wastewater generated from Taguchi OA design.
Results and discussion
Heavy metals in collected sample
Heavy metals characterization was carried with the use of an Atomic Adsorption Spectroscopy (ASS), it relies on the absorption of light by atoms in the ground state, which occurs at characteristic wavelengths for each element. There were ten heavy metals present in the wastewater samples, and they are shown Table 4, alongside the comparison of World Health Organization (WHO) wastewater disposal standards for the specific heavy metals. From the result obtained, the concentrations of As, Ca, Fe and Zn were less than the permissible concentration stated by the WHO. However, the concentrations of Cd, Cu, Ni, Cr, Pb and Mn were above the WHO permissible limit suggesting their removal from the hospital wastewater is very imperative before the disposal into the environment. Generally, this hospital wastewater is not allowed to be disposed into the environment until adequate treatment because many of the heavy metals present were above the WHO permissible limit.
Physiochemical properties of collected sample
Physiochemical properties refer to the properties of a substance that can be measured or observed and are related to both its physical and chemical characteristics. There are various physiochemical properties, but only a few were considered and they include; turbidity, salinity, conductivity, pH and the total dissolved solids (TDS). These properties were determined through their respective meters. The pH was checked using OAKION pH meter (S/N 2202625, Eutech Instruments, Singapore) and turbidity through the turbidity meter. The salinity, conductivity and TDS were checked using the Mettler Toledo, an equipment that has the ability to check for these properties using its probe. Table 5 shows the results for the physiochemical property of the wastewater sample collected from ABUAD multisystem hospital. The results revealed that the total dissolved solids and the conductivity of the hospital wastewater were less than the permissible limit set by the WHO. However, the turbidity and pH of the hospital wastewater were higher than the WHO permissible limit suggesting that the treatment of the wastewater is imperative before disposal.
Characterization of adsorbent
Scanning electron microscopy
Figure 1a depicts the adsorbent surface before adsorption. The morphological surface revealed the presence of unequally distributed particles with rough surfaces characterized with large pore openings to accommodate the adsorption of pollutants present in the hospital wastewater examined. This observation could be attributed to the chemical modification of the banana peels using sulfuric acid and sodium hydroxide, which helps in breaking down the long cellulose chains of the banana peel particles. Also, the reaction between the active functional groups present in the banana peel particles and the modification reagents enhances the increase in the pore openings to treat the hospital wastewater (Popoola 2023a). The banana peel particles were pre-hydrolyzed via the modification before being applied as adsorbent for the treatment process. After the treatment of the hospital wastewater via the adsorption process, the SEM monograph (Fig. 1b) depicts the presence of tiny particles mixed with few unevenly distributed large particles resulting from the dissolution of the banana peel particles in the hospital wastewater. A reduction in the large pore openings, previously observed before the treatment, was noticed. This could be attributed to the filling up of these large openings by the pollutants present in the hospital wastewater. Also, the presence of saturated solution of the hospital wastewater in the banana peel particles could be noticed after the treatment.
Fourier-transform infrared spectroscopy (FTIR)
The FTIR analysis was carried out using the transmission method and the result obtained for adsorbent before and after adsorption process are shown in Fig. 2. FTIR (Fourier Transform Infrared) spectroscopy is a technique used to analyze the chemical groups and bonds present in a sample by studying the peaks observed in its spectrum. The provided FTIR graph shows peaks at specific wavenumbers (cm−1) before and after the adsorption process, along with their corresponding transmittance values (%). Before adsorption (Fig. 2a), the peaks at 3300 cm−1, 23,504 cm−1, 1635 cm−1, and 1504 cm−1 were noticed suggesting the presence of hydroxyl groups, carbon–nitrogen or nitrogen–nitrogen triple bonds, carbon–carbon double bonds, and aromatic compounds, respectively. Shift in peaks was observed after the adsorption (Fig. 2b) to 3325 cm−1, 1635 cm−1, 1414 cm−1, and 1031 cm−1 indicating adsorption has taken place. In summary, the changes in the FTIR peaks after the adsorption process indicate chemical interactions and modifications on the surface of the adsorbent. The appearance of peaks related to carbonyl groups, amine groups, and oxygen-containing functional groups suggests the involvement of these groups in the adsorption process, potentially leading to the formation of adsorbate–adsorbent complexes or chemical reactions between them.
Batch treatment using Taguchi orthogonal array
Percentage removal of heavy metals in hospital wastewater
The nine experimental runs generated from the Taguchi orthogonal array used in the design of experiment (Table 3) for the treatment of hospital wastewater using chemically modified banana peels was adopted to generate the results presented in Table 6 showing the percentage removal of heavy metals in the sample after treatment via adsorption process. The heavy metal percentage removed was determined using Eq. (1). On an average across all the experimental runs, Pb and Mn have the highest and lowest percentage removal, respectively, out of all the heavy metals present in the hospital wastewater. An average of 80.67% and 24% were, respectively, recorded for Pb and Mn. This strongly suggests that the chemically modified banana peel has strong adsorption affinity for Pb among the heavy metals. The result presented by Afolabi et al. (2022) was similar proving natural banana peel to exhibit high adsorption capacity for Pb2+ in its binary mixture with Cu2+ in solution. Also, from all the experimental runs, run 5 conducted at temperature, adsorbent dosage, mixing rate and contact time of 30 °C, 3 g, 700 rpm and 60 min, respectively, revealed the highest removal efficiencies for the heavy metals except As, Mn and Zn. The percentage of Cd, Fe, Cu, Ni, Cr and Pb removed were 79.5%, 87.9%, 56.9%, 89.0%, 88.5% and 93.5%, respectively.
For As, 100% removal was observed by the chemically modified banana peel at experimental run 9 conducted at temperature, adsorbent dosage, mixing rate and contact time of 30 °C, 1 g, 125 rpm and 30 min, respectively. The chemically modified banana peel revealed 100% removal for Mn at experimental runs 3 and 7 conducted at temperature, adsorbent dosage, mixing rate and contact time of 40 °C, 3 g, 125 rpm and 45 min; and 50 °C, 1 g, 700 rpm and 45 min; respectively. For Zn, 100% removal was observed at experimental run 4 conducted at temperature, adsorbent dosage, mixing rate and contact time of 40 °C, 3 g, 124 rpm and 45 min, respectively. The variations in the experimental runs under which maximum percentage removal was recorded could be attributed to the favorable operating conditions that is suitable for the removal of each of the heavy metals from the hospital wastewater.
where Co = Initial concentration of heavy metals (ppm) and Cf = Final concentration of heavy metals (ppm).
Mathematical model development and correlation coefficient
This study examines four parameters at three levels: temperature (30, 40 and 50 °C), contact time (30, 45 and 60 min), mixing rate (125, 350 and 700 rpm) and adsorbent dosage (1, 2 and 3 g) using Taguchi OA of design expert 12. Consideration was given to Ni and Cu to test the effectiveness of the adopted Taguchi OA methodology in mathematical model development for the prediction of heavy metals removal from the hospital wastewater using chemically modified banana peel. Besides, Ni and Cu were the heavy metals that exhibited the most significant level amidst the responses. The predictive model equations to determine the percentage of Ni and Cu removed from the wastewater are presented as Eqs. (2 and 3), respectively.
where A, B, C and D represent temperature, contact time, mixing rate and adsorbent dosage respectively. The levels of the corresponding model terms are indicated in the square bracket.
The correlation plots between the experimental and predicted values of Ni and Cu responses are shown in Fig. 3 with correlation coefficient (R2) of 0.9537 and 0.9707, respectively. It shows that the experimental and predicted values have a strong connection. The outcome suggested that the model was best suited to forecast the process.
Statistical analysis
The analysis of variance (ANOVA) of the Taguchi orthogonal array was used as the statistical tool to analyze the responses. However, out of all the responses, Cu and Ni were the most significant.
Copper (Cu)
The analysis of variance, ANOVA for copper (Cu), is shown in Table 7. The model, including all the factors being investigated, shows a significant effect on Cu removal. The F-value of 130.687 and the very low p value of 0.0076 indicate a highly significant relationship between the factors and the response. The temperature, contact time, dosage and mixing rate have F-value of 127.93, 142.07, 371.37 and 41.76, respectively. Their respective p value is 0.0092, 0.0071, 0.0027and 0.0784 which is below the significance level of 0.05. This suggests that the parameters have significant effect on Cu removal.
Table 8 presents the ANOVA fit statistics which gives useful information about the model’s quality for Cu removal. The 0.814 standard deviation (Std. Dev.) number shows the variability of observed data points around the mean, with a lower value indicating a more precise and dependable model. The coefficient of correlation (R2) of 0.997 indicates that the independent variables can explain roughly 99.7% of the variation in Cu removal, indicating a strong correlation and a well-fitting model. The adjusted R2 value of 0.990 takes the number of independent variables into account, providing a conservative estimate of model fit and lowering the danger of overfitting. The estimated R2 of 0.948 demonstrates the model’s accuracy in predicting Cu elimination in the future. A coefficient of variation (C.V.%) of 1.800 represents the data’s relative variability in comparison with the mean, with a lower number indicating greater precision. The adequate precision value of 31.174 indicates that the model can forecast Cu removal with increased precision. Overall, the Cu removal model has outstanding goodness of fit, precision, and dependability. The high R2 and adjusted R2 values indicate a strong association between the independent and response variables; while, the low standard deviation and C.V.% values verify the model’s correctness.
Nickel (Ni)
The analysis of variance, ANOVA for nickel (Ni), is shown in Table 9. The model, including all the factors being investigated, shows a significant effect on Ni removal. The F-value of 78.85 and the low p value of 0.0126 indicate a highly significant relationship between the factors and the response. The temperature, contact time, dosage and mixing rate have F-value of 38.30, 23.42, 211.82 and 35.30, respectively. Their respective p value is 0.0333, 0.0409, 0.0047 and 0.0353 which is below the significance level of 0.05. This suggests that the parameters have significant effect on Ni removal.
Table 10 presents the ANOVA fit statistics for Ni removal. The standard deviation (Std. Dev.) value of 1.570 indicates the variability of the observed data points around the mean value. A lower standard deviation suggests that the data points are closely clustered around the mean, indicating a more precise and reliable model. The coefficient of correlation (R2) value of 0.996 indicates that approximately 99.6% of the variation in the response variable (removal of Ni) can be explained by the independent variables in the model. This high R2 value suggests a strong correlation between the independent variables and the removal of Ni, indicating that the model provides a good fit to the data. The adjusted R2 value of 0.983 takes into account the number of independent variables in the model. It provides a more conservative estimate of the model’s goodness of fit by penalizing the addition of unnecessary variables. A higher adjusted R2 value suggests that the model is less likely to be overfitting the data. The predicted R2 value of 0.915 indicates the reliability of the model in predicting future observations. It suggests that approximately 91.5% of the variation in the removal of Ni for new data points can be explained by the model. The coefficient of variation (C.V. %) value of 2.115 indicates the relative variability of the data compared to the mean value. A lower C.V. % suggests that the data points have less variation relative to the mean, indicating a more precise and reliable model. The adequacy precision value of 24.597 reflects the signal-to-noise ratio in the model. A higher value suggests that the model is more precise in predicting the removal of Ni.
Optimum point prediction
The predicted optimum conditions for the hospital wastewater treatment were generated from the Taguchi OA of design expert. For the optimum point prediction, consideration was given to the heavy metals with highest percentage removal and other physiochemical properties. Figure 4 revealed the optimum conditions for the treatment process to exist at a temperature of 50 °C (Fig. 4a), contact time of 45 min (Fig. 4b), adsorbent dosage of 1 g (Fig. 4c) and mixing rate of 125 rpm (Fig. 4d). Figure 5 represents the desirability of the responses and the percentage removal for each of the heavy metals and physicochemical properties at the optimum point. The values of the percentage removal of Cd, Fe, Cu, Ni, Cr, Pb, and Zn were 64.3%, 80.5%, 51.4%, 82.3%, 72.4%, 86.6%, and 72.1%, respectively. The conductivity, pH, TDS and turbidity value at the optimum conditions were shown to be 20.57 mS/cm, 6.8, 10.16 mg/L and 86.31 NTU, respectively. The pH value was optimized to be selected within a range of 6.5 and 8.5.
Conclusion
In this study, chemically modified banana peel has shown promising potential as an effective adsorbent for the hospital wastewater treatment via the removal of pollutants such as heavy metals and lowering the physicochemical properties to the permissible limit of WHO. The experimental design for optimization was developed using Taguchi OA design considering temperature, contact time, dosage and mixing rate at three different levels as process variables. The percentage of Cd, Fe, Cu, Ni, Cr and Pb removed were 79.5%, 87.9%, 56.9%, 89.0%, 88.5% and 93.5%, respectively, at temperature, adsorbent dosage, mixing rate and contact time of 30 °C, 3 g, 700 rpm and 60 min, respectively. The physicochemical properties remain within the acceptable WHO range except the turbidity. At optimum point, the percentage removal of Cd, Fe, Cu, Ni, Cr, Pb, and Zn were 64.3%, 80.5%, 51.4%, 82.3%, 72.4%, 86.6%, and 72.1%, respectively; while, the conductivity, pH, TDS and turbidity value were, respectively, 20.57 mS/cm, 6.8, 10.16 mg/L and 86.31 NTU. At this point, the predicted values for temperature, contact time, dosage and mixing rate were 50 °C, 45 min, 1 g and 125 rpm, respectively. Characterization revealed the existence of active functional groups on the adsorbent surface enhancing the hospital wastewater treatment and change in its morphological structure after the treatment was noticed. In conclusion, the modified banana peel was effective for the treatment of hospital wastewater.
Data availability
The data used to support the findings of this study are included within the article.
References
Abdulfatai J, Saka AA, Afolabi AS, Micheal O (2013) Development of adsorbent from banana peel for wastewater treatment. Appl Mech Mater 248:310–315
Adeyi AA, Uzoukwu MC, Popoola LT, Ogunyemi AT, Hamisu A (2022) Equilibrium and kinetic analysis on cadmium ion sequestration from aqueous environment by impregnated chicken feather alkaline biosorbent. Int J Eng Res Afr 60:15–28
Adeyi AA, Giwa A, Abdullah LC, Popoola LT, Jamil SNAM, Lala MA (2023b) Sequestration of diclofenac and amoxycillin pharmaceutical compounds by thiourea modified poly(acrylonitrile-co-acrylic acid): parametric optimisation, kinetic and isotherm studies. Int J Environ Anal Chem. https://doi.org/10.6084/m9.figshare.23796992.v1
Adeyi AA, Bitrus NK, Abdullah LC, Popoola LT, Omotara OO, Saber SEM (2023a) Effective sequestration of levofloxacin from wastewater by biochar-supported manganese dioxide composite: experimental study and modelling analyses. Environ Eng Res 28(1):210512
Afolabi FO, Musonge P, Bakare BF (2021) Bio-sorption of copper and lead ions in single and binary systems onto banana peels. Cogent Eng 8(1):1886730
Afolabi FO, Musonge P, Bakare BF (2022) Adsorption of copper and lead ions in a binary system onto orange peels: optimization, equilibrium, and kinetic study. Sustainability 14:10860
Annadurai G, Juang RS, Lee DJ (2003) Adsorption of heavy metals from water using banana and orange peels. Water Sci Technol 47(1):185–190
Arifiyana D, Devianti VA (2021) Optimization of pH and contact time adsorption of banana peels as adsorbent of Co(II) and Ni(II) from liquid solutions. AIP Conf Proc 2330:070007. https://doi.org/10.1063/5.0043112
Arunakumara K, Walpola BC, Yoon MH (2013) Banana peel: a green solution for metal removal from contaminated waters. Korean J Environ Agric 32(2):108–116
Asfaw T (2018) Review on hospital wastewater as a source of emerging drug resistance pathogens. J Res Environ Sci Toxicol 7(2):7–52
Chen H, Li W, Wang J, Xu H, Liu Y, Zhang Z, Li Y, Zhang Y (2019) Adsorption of cadmium and lead ions by phosphoric acid-modified biochar generated from chicken feather: selective adsorption and influence of dissolved organic matter. Bioresour Technol 292:121948
El-Sayed HEM, El-Sayed MMH (2014) Assessment of food processing and pharmaceutical industrial wastes as potential biosorbents: a review. BioMed Res Int. https://doi.org/10.1155/2014/146769
Fita G, Djakba R, Mouhamadou S, Duc M, Rao S, Popoola LT, Harouna M, Benoit LB (2023a) Adsorptive efficiency of hull-based activated carbon toward copper ions (Cu2+) removal from aqueous solution: Kinetics, modelling and statistical analysis. Diam Relat Mater 139:110421
Fita G, Djakba R, Mouhamadou S, Duc M, Rao S, Popoola LT, Harouna M, Benoit LB (2023b) Adsorptive efficiency of hull-based activated carbon toward copper ions (Cu2+) removal from aqueous solution: kinetics, modelling and statistical analysis. Diam Relat Mater 139:110421
Frédéric O, Yves P (2014) Pharmaceuticals in hospital wastewater: their ecotoxicity and contribution to the environmental hazard of the effluent. Chemosphere 115(1):31–39
Gautam S, Khan S (2016) Removal of methylene blue from waste water using banana peel as absorbent. Int J Sci Environ 5(5):3230–3236
Gomes S, Vieira B, Barbosa C, Pinheiro R (2020) Evaluation of mature banana peel flour on physical, chemical and texture properties of a gluten free Rissol. J Food Process Preserv 46:e14441
Gunatilake SK (2015) Methods of removing heavy metals from industrial wastewater. Methods 1(1):12–18
Hocaoglu SM, Celebi MD, Basturk I, Partal R (2021) Treatment-based hospital wastewater characterization and fractionation of pollutants. J Water Process Eng 43:102205
Huzaisham NA, Marsi N, Rus AZM, Masrol SR, Mahmood S, Main NM, Fodzi MHM, Singam RAT (2020) Application of waste banana peels for wastewater treatment: a Review. J Comput Theor Nanosci 17(2):596–602
Jin Q, Wang Z, Feng Y, Kim YT, Stewart AC, O’Keefe SF, Neilson AP, He Z, Huang H (2020) Grape pomace and its secondary waste management: biochar production for a broad range of lead (Pb) removal from water. Environ Res 186:109442
Khan MT, Shah IA, Ihsanullah I, Naushad M, Ali S, Shah SHA, Mohammad AW (2021) Hospital wastewater as a source of environmental contamination: an overview of management practices, environmental risks and treatment processes. J Water Process Eng 41:101990
Lala MA, Ntamu TE, Adesina OA, Popoola LT, Yusuff AS, Adeyi AA (2023) Adsorption of hexavalent chromium from aqueous solution using cationic modified rice husk: parametric optimization via Taguchi design approach. Sci Afr 20:e01633
Lee ME, Park JH, Chung JW (2019) Comparison of the lead and copper adsorption capacities of plant source materials and their biochars. J Environ Manag 236:118–124
Leong S (2018) Adsorption of heavy metals using banana peels in wastewater treatment. Dergipark Org Tr 2:312–317
Li C, Yu Y, Zhang Q, Zhong H, Wang S (2020) Removal of ammonium from aqueous solutions using zeolite synthesized from electrolytic manganese residue. Int J Chem Eng 2020:8818455
Macías-García A, García-Sanz-Calcedo J, Carrasco-Amador JP, Segura-Cruz R (2019) Adsorption of paracetamol in hospital wastewater through activated carbon filters. Sustainability 11(9):1–11
Majumder A, Gupta AK, Ghosal PS, Varma M (2021) A review on hospital wastewater treatment: a special emphasis on occurrence and removal of pharmaceutically active compounds, resistant microorganisms and SARS-CoV-2. J Environ Chem Eng 9(2):1–44
Mohamed RM, Hashim N, Abdullah S, Abdullah N, Mohamed A, Asshaary Daud MA, Aidil Muzakkar KF (2020) Adsorption of heavy metals on banana peel bioadsorbent. J Phys: Conf Ser 1532(1):012014. https://doi.org/10.1088/1742-6596/1532/1/012014
Mompelat S, Le Bot B, Thomas O (2009) Occurrence and fate of pharmaceutical products and by-products, from resource to drinking water. Environ Int 35(5):803–814
Nagarajaiah SB, Prakash J (2011) Chemical composition and antioxidant potential of peels from three varieties of banana. As J Food Ag-Ind 4(01):31–46
Oliveira GA, Gevaerd A, Mangrich AS, Marcolino-Junior LH, Bergamini MF (2021) Biochar obtained from spent coffee grounds: evaluation of adsorption properties and its application in a voltammetric sensor for lead (II) ions. Microchem J 165:106114
Paulus GK, Hornstra LM, Alygizakis N, Slobodnik J, Thomaidis N, Medema G (2019) The impact of on-site hospital wastewater treatment on the downstream communal wastewater system in terms of antibiotics and antibiotic resistance genes. Int J Hyg Environ Health 222:635–644
Perini JAL, Tonetti AL, Vidal C, Montagner CC, Pupo Nogueira RF (2018) Simultaneous degradation of ciprofloxacin, amoxicillin, sulfathiazole and sulfamethazine, and disinfection of hospital effluent after biological treatment via photo-Fenton process under ultraviolet germicidal irradiation. Appl Catal B Environ 224:761–771
Popoola LT (2019a) Characterization and adsorptive behaviour of snail shell-rice husk (SS-RH) calcined particles (CPs) towards cationic dye. Heliyon 5:e01153
Popoola LT (2019b) Nano-magnetic walnut shell-rice husk for Cd(II) sorption: design and optimization using artificial intelligence and design expert. Heliyon 5:e02381
Popoola LT (2020) Tetracycline and sulfamethoxazole adsorption onto nanomagnetic walnut shell-rice husk: isotherm, kinetic, mechanistic and thermodynamic studies. Int J Environ Anal Chem 100(9):1021–1043
Popoola LT (2023a) Efficient Cr(VI) sequestration from aqueous solution by chemically modified Garcinia kola hull particles: characterization, isotherm, kinetic and thermodynamic studies. Environ Sci Pollut Res. https://doi.org/10.1007/s11356-023-29848-0
Popoola LT (2023b) Taguchi parametric optimization and cost analysis of hexavalent chromium sequestration from aqueous solution by NaOH-modified Garcinia kola hull particles. Environ Health Insights 17:1–11
Popoola LT, Aderibigbe TA, Yusuff AS, Munir MM (2018) Brilliant green dye adsorption onto composite snail shell –rice husk: adsorption isotherm, kinetic, mechanistic and thermodynamic analysis. Environ Qual Manage 28:2–3. https://doi.org/10.1002/tqem.21597
Popoola LT, Yusuff AS, Aderibigbe TA (2019) Assessment of natural groundwater physico-chemical properties in major industrial and residential locations of Lagos metropolis. Appl Water Sci 9:191. https://doi.org/10.1007/s13201-019-1073-y
Popoola LT, Yusuff AS, Adeyi AA, Omotara OO (2022) Adsorptive removal of heavy metals from oil well produced water using citrullus lanatus peel: characterization and optimization. S Afr J Chem Eng 39:19–27
Renita AA, Kumar PS, Srinivas S, Priyadharshini S, Karthika M (2017) A review on analytical methods and treatment techniques of pharmaceutical wastewater. Desalin Water Treat 87:160–178
Stalder T, Barraud O, Jové T, Casellas M, Gaschet M, Dagot C, Ploy MC (2014) Quantitative and qualitative impact of hospital effluent on dissemination of the integrin pool. ISME J 8(4):768–777
Syed-Hassan SSA, Zaini MSM (2016) Optimization of the preparation of activated carbon from palm kernel shell for methane adsorption using Taguchi orthogonal array design. Korean J Chem Eng 33(8):2502–2512
Wielens Becker R, Ibanez M, Cuervo Lumbaque E, Luís Wilde M, Flores Da Rosa T, Hern´andez F, Sirtori C (2020) Investigation of pharmaceuticals and their metabolites in Brazilian hospital wastewater by LC-QTOF MS screening combined with a preliminary exposure and in silico risk assessment. Sci Total Environ 699:1–14
Williams M, Kookana RS (2018) Fate and behavior of environmental contaminants arising from health-care provision. Health care and environmental contamination. Elsevier, Amsterdam. https://doi.org/10.1016/b978-0-444-63857-1.00003-6
Yusuff AS (2019) Optimization of the preparation conditions of an eggshell/anthill composite adsorbent using a central composite design. J Chem Technol Metall 54(6):1202–1214
Yusuff AS, Ajayi OA, Popoola LT (2021a) Application of Taguchi design approach to parametric optimization of adsorption of crystal violet dye by activated carbon from poultry litter. Sci Afr 13:e00850
Yusuff AS, Popoola LT, Anochie V (2021b) Utilization of agricultural waste adsorbent for the removal of lead ions from aqueous solutions. Acta Polytech 61(4):1–9
Yusuff AS, Popoola LT, Igbafe AI (2022) Response surface modeling and optimization of hexavalent chromium adsorption onto eucalyptus tree bark-derived pristine and chemically-modified biochar. Chem Eng Res Des 182:592–603
Zaini HM, Roslan J, Saallah S, Munsu E, Sulaiman NS, Pindi W (2022) Banana peels as a bioactive ingredient and its potential application in the food industry. J Funct Foods 92:105054
Zekavat SR, Raouf F, Talesh SSA (2020) Simultaneous adsorption of Cu2+ and Cr(VI) using HDTMA-modified zeolite: Isotherm, kinetic, mechanism, and thermodynamic studies. Water Sci Technol 82(9):1808–1824
Zhou N, Chen H, Xi J, Yao D, Zhou Z, Tian Y, Lu X (2017) Biochars with excellent Pb (II) adsorption property produced from fresh and dehydrated banana peels via hydrothermal carbonization. Bioresour Technol 232:204–210
Acknowledgements
The support given by Afe Babalola University, Ado-Ekiti, Nigeria in providing enabling environment to execute this research work is acknowledged. The contribution of Mr. Edoh Paul of the Water Treatment Laboratory, Civil Engineering Department, Afe Babalola University on the physicochemical characterization is appreciated.
Funding
No financial support in form of funding was received for the execution of this research work.
Author information
Authors and Affiliations
Corresponding authors
Ethics declarations
Conflict of interest
The author declares no conflict of interest in terms of financial or personal nature.
Additional information
Publisher's Note
Springer Nature remains neutral with regard to jurisdictional claims in published maps and institutional affiliations.
Rights and permissions
Open Access This article is licensed under a Creative Commons Attribution 4.0 International License, which permits use, sharing, adaptation, distribution and reproduction in any medium or format, as long as you give appropriate credit to the original author(s) and the source, provide a link to the Creative Commons licence, and indicate if changes were made. The images or other third party material in this article are included in the article's Creative Commons licence, unless indicated otherwise in a credit line to the material. If material is not included in the article's Creative Commons licence and your intended use is not permitted by statutory regulation or exceeds the permitted use, you will need to obtain permission directly from the copyright holder. To view a copy of this licence, visit http://creativecommons.org/licenses/by/4.0/.
About this article
Cite this article
Popoola, L.T., Yusuff, A.S., Taura, U. et al. Wastewater treatment from a typical multisystem hospital using chemically modified banana peels: Taguchi parametric optimization and characterization. Appl Water Sci 14, 112 (2024). https://doi.org/10.1007/s13201-024-02173-8
Received:
Accepted:
Published:
DOI: https://doi.org/10.1007/s13201-024-02173-8